Welcome to this edition of thought leadership, where you can discover the latest eCommerce insights direct from industry experts. This month, Adam Hjort, VP of Customer Success for Loop54, discusses the different methods recommendations engines use along with the drawbacks each presents.
Personalization has been a buzzword for quite some time in the eCommerce space, similar to past buzzwords such as “Big Data” or “AI”. However, it’s also very clear that the definitions of personalization are very different depending on who you are asking about them. The same goes for recommendations. What’s for certain, however, is that recommendations are here to stay and that they have a huge impact on the bottom line of any eCommerce business.
It is not all that strange that definitions of recommendations vary because they do come in different forms. Below is a list of what we see as recommendations:
Similar products – These are products that are very similar to other products. These are typically shown on product detail pages and the main purpose of these is to keep the customer engaged when the particular product they have clicked on is not to their liking. The logic is this: if the clicked product initially sparked interest, maybe similar products could keep the visitor engaged at the website.
Popular products – These are products that are, on an aggregated level, more popular than other products. The popularity can be measured in different ways, but the most common ones are clicks, views or purchases. These are used to increase conversions of an eCommerce website by showing which products other visitors engage with.
Complementary products – These are products that complement the product engaged with. Examples of these could be accessories to a “main” product, such as shoelaces to a boot or maybe a paddle to a kayak. The main purpose of complementary products is to increase the average order value (AOV) by making the visitor purchase more products than the ones they have already bought. There are different ways to populate complementary products which range from AI-based context awareness and image recognition to manual handling and historical purchase patterns.
Basket recommendations – These are recommendations that are based on the visitor’s actual cart (or basket). Similar to complementary products, the main purpose of basket recommendations is to increase AOV. Furthermore, this is the only type of recommendation that is personalized by default (whereas the other ones can be personalized).
So how do recommendation engines know which product to recommend?
First of all, we need to reach common ground when it comes to what a good recommendation actually is. The reason for this, as I mentioned earlier, is that the definition might vary depending on who you are asking. For us, a good recommendation is a recommendation that proves your hypothesis, which is, by most definitions, the one that provides the highest conversion rate. Please note, however, that by just looking at the conversion rate, you might actually end up showing recommendations that are converting very well but might just moderately affect PSV (per session value) or AOV. The reason behind this is that there is a very high correlation between the price of the recommendation and the actual conversion rate. The cheaper the product, the higher the conversion rate. Therefore, we recommend you focus on the per session value, which compensates for this (PSV = revenue ÷ sessions).
So, now that we have found a common definition of what a good recommendation actually is, how do we choose which product to recommend? Most recommendation engines do this on different levels, but almost all have in common that they look at historical purchasing data to understand which products are often bought together. At the rudimentary level, this approach requires no AI or understanding of the actual relationship between products. This approach also works very well for 10% of your product assortment.
For the 90% remaining assortment, there will be very weak connections between products. This is due to the fact that buying behavior varies from session to session but also because your product assortment is ever-changing. Old products are discontinued and new ones are being launched, which either removes connections or has no history to start with. To handle this, let’s call it a data problem, we can find different approaches. Some engines use image recognition technology to understand which products are similar and build a context-awareness based on this. Other engines use NLP (natural language processing) to get a better understanding of products and how they relate to each other, and some do nothing to understand the context but rather rely on human intelligence to create good recommendations.
Image recognition
This technology has made huge technological advancements in the last few years and today provides this functionality in platforms such as Google photos to group or tag photos. The technology is in many cases very good and provides a great value to classifying and organizing attributes of photos. When this technology is used for product classification, however, there are some drawbacks.
First of all, image recognition is great at classifying disparate images with varying properties (such as the Not Hotdog app) but does less well at finding granular differences between products. Therefore, it works well at identifying, for example, dresses or skirts that have a similar appearance but has no idea that a coat rack and household paper holder might have a very similar appearance. For department stores, this could have some serious implications for the recommendations shown.
A coat rack or household paper holder? Image recognition is not always able to accurately identify product similarities and differences.
Natural language processing
Another way to understand context is by trying to understand how products relate to each other based on the description. This approach has similar drawbacks as image recognition since it does only capture a part of the actual product. A way to exemplify this is to look at a product attribute such as color. Some products are multi-colored and in many product feeds, this is the actual value of the attribute. A human would understand that the product has many colors, but it tells nothing about what it actually looks like. The obvious drawback of this method is that some products will not really have any context and recommendations might become very irrelevant.
Manual selection of recommendations
This method is probably the best one in terms of relevance. Humans are great at understanding which products fit together, maybe best exemplified by visual merchandisers. The task might seem like a simple one if your assortment consists of 500 products, but what if it has 10,000 or maybe 100,000?
Just updating these recommendations on a regular basis easily becomes a full-time job. Furthermore, this method relies solely on the discretion of the merchandiser doing it and it also makes it impossible to personalize the experience for each and every visitor based on either historical purchasing data or session intent.
This Venn diagram illustrates the relationship and overlap between the different types of recommendations.
Context unaware recommendations
A fourth option is to ignore the concept of context and instead base the recommendations on other variables such as historical purchasing data. This method is actually not that unusual and can, combined with manual merchandising, compete with other automated methods such as image recognition. The benefit of looking at historical purchasing data is that the products that are recommended using this method have a proven conversion propensity and the risk is showing completely irrelevant recommendations. A grill could for example be recommended on the product page for a pencil.
Understanding context by looking at visitor behavior
There is, however, another option. What we have learned from working with context awareness and relevance for 10 years is that GOLEM (our contextual aware relevance algorithm) can solve the above drawbacks in a very effective way. Without digging too deep into the technical characteristics of GOLEM and our AI, what we’re doing is understanding the context by connecting product attributes to neurons. These neurons are then exposed to actions that your visitors are taking when they visit your website which teaches the engine which products are similar to each other, and which are far apart.
This unique method of understanding context creates an extraordinary capability of selecting the most relevant recommendations available, without the drawbacks of image recognition, NLP, or manual merchandising. Furthermore, the method is combinable with all the above methods to create even higher relevance and conversion propensity.
This method is also compatible with personalization, both with session intent and historical preferences such as brand affinity, price sensitivity, or any type of preference you’d like to prioritize. The method also supports streaming data, which basically means that any new product you’d choose to release will instantly be incorporated into the model without having any historical data on it, making it even more powerful for businesses that have a lot of seasonality built in their assortment, such as the fashion industry.
Which method have you been using and what are your key takeaways? Let us hear your thoughts, drop us a line below!
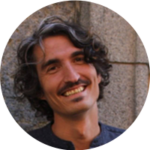
About the author
Adam Hjort is the VP of Customer Success at Loop54. He is an experienced leader within SaaS, been working within the industry for 10 years, with a background in Sales, Product Management and COO. Lecturer and speaker in AI, eCommerce and sales.